Introduction
Correlation is a fundamental statistical concept that quantifies the strength and direction of a relationship between two or more variables. It is a crucial tool in data analysis, helping us identify patterns, make predictions, and gain insights into various fields, including science, finance, social sciences, and more. In this article, we will explore the concept of correlation, its types, how to measure it, and its practical applications.
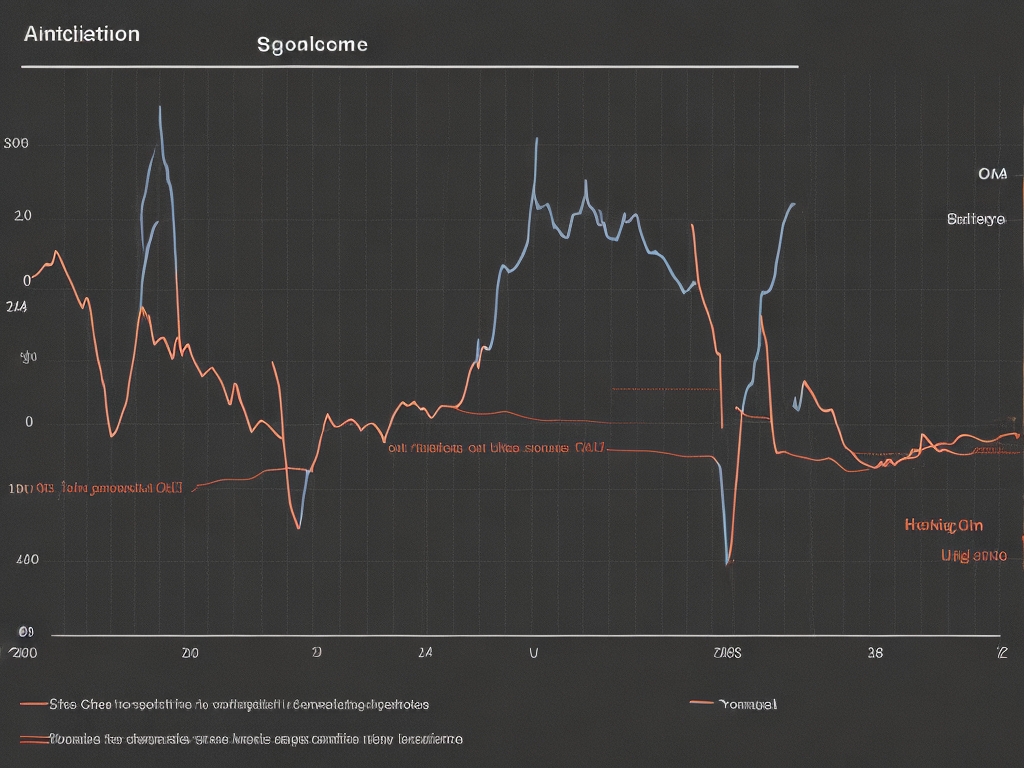
What is Correlation?
Correlation refers to the statistical association or relationship between two or more variables. When variables are correlated, changes in one variable tend to be associated with changes in another. Correlation does not imply causation, meaning that just because two variables are correlated does not mean that one causes the other.
Types of Correlation
There are several types of correlation, with the most common being:
- Positive Correlation: In a positive correlation, as one variable increases, the other also tends to increase. Conversely, as one decreases, the other tends to decrease. This is often represented as a positive correlation coefficient.
- Negative Correlation: In a negative correlation, as one variable increases, the other tends to decrease, and vice versa. This is represented by a negative correlation coefficient.
- Zero Correlation: When there is no apparent relationship between two variables, they are said to have zero correlation, often represented by a correlation coefficient close to zero.
Measuring Correlation
The strength and direction of correlation between two variables are typically measured using correlation coefficients. The most common correlation coefficient is the Pearson correlation coefficient (also known as Pearson’s r), which quantifies the linear relationship between variables. The Pearson correlation coefficient can range from -1 to 1:
- A coefficient of 1 indicates a perfect positive correlation.
- A coefficient of -1 indicates a perfect negative correlation.
- A coefficient of 0 indicates no linear correlation.
Other correlation coefficients include Spearman’s rank correlation coefficient, Kendall’s Tau, and point-biserial correlation, each designed for specific types of data or relationships.
Practical Applications of Correlation
Correlation is widely used in various fields and applications:
- Finance: Correlation analysis helps investors and portfolio managers diversify their investments by understanding how different assets are correlated. It plays a crucial role in risk management and asset allocation.
- Medicine: In medical research, correlation analysis can be used to identify relationships between variables, such as the correlation between a certain lifestyle factor and a health condition.
- Economics: Economists use correlation to study the relationships between economic indicators, helping to make predictions about economic trends.
- Marketing: Marketers analyze the correlation between advertising spending and sales to assess the effectiveness of marketing campaigns.
- Environmental Science: Correlation is used to study the impact of environmental factors on ecosystems and wildlife populations.
The Importance of Interpretation
While correlation can provide valuable insights, it’s essential to interpret the results carefully. Correlation does not imply causation, so it’s crucial to avoid making causal claims based solely on correlation. Additionally, outliers and confounding variables can influence correlation results, so a thorough analysis is necessary.
Conclusion
Correlation is a vital statistical concept that helps us understand the relationships between variables in various fields. Whether you are analyzing financial data, conducting medical research, or studying economic trends, correlation analysis provides valuable insights into the connections between variables. When used correctly and with proper interpretation, correlation can be a powerful tool for data-driven decision-making and research.